There is an ever increasing number of websites dedicated to aiding in analytics and investing in Lending Club and Prosper. In part two of this comprehensive two-part series (click here for Part 1) we will look at all of the popular tools that investors use today (there are ten in all) in order to backtest strategies, automate their investments and analyze data. Analytics and data has been a large part of p2p lending from the start and investors are always looking for an advantage to potentially maximize returns. Investment automation allows for a much better investment experience versus selecting notes on the platform by hand.
BlueVestment specializes in automation strictly for LendingClub. Through BlueVestment, users are able to automate investing in notes using credit models from P2P-Picks. P2P-Picks has created their own models based on historic data from Lending Club and Prosper. Investing using either the Profit Maxmizer or Loss Minimizer models is a hands off method of investing in Lending Club. As new data becomes available, the models are updated accordingly to achieve the results of the respective model.
Users can also opt to create their own basic filter criteria using the 22 attributes available to them. In addition, users can create advanced filters using the node builder. This allows for a customized investing experience with different operators as shown below.
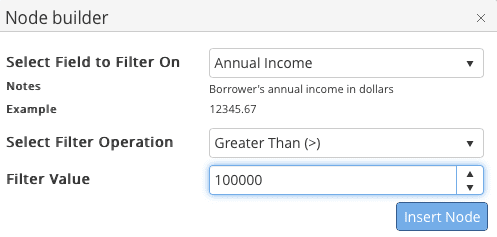

BlueVestment also has a high level dashboard which includes investment amount, available loan distribution by grade and total loan availability by grade. BlueVestment is a completely free service.
PeerTrader currently offers automation and charting specifically for Prosper with Lending Club integration coming soon. They are currently in open beta and will be free for anyone to use until they officially launch. As of 2015, they are now an SEC Registered Investment Adviser which makes them one of two public retail tools that are an RIA. PeerTrader has just completed their integration with P2P-Picks, which is publicly available when you create a PeerTrader account.
PeerTrader also offers streaming charts which are interesting to watch during the release times. Among the charts, you can see Total Investable Volume and Number of Active Listings as shown in the screenshot below.

The screenshot below shows an example of how to setup an auto-invest with PeerTrader. They currently offer 19 filters to customize your auto invest.
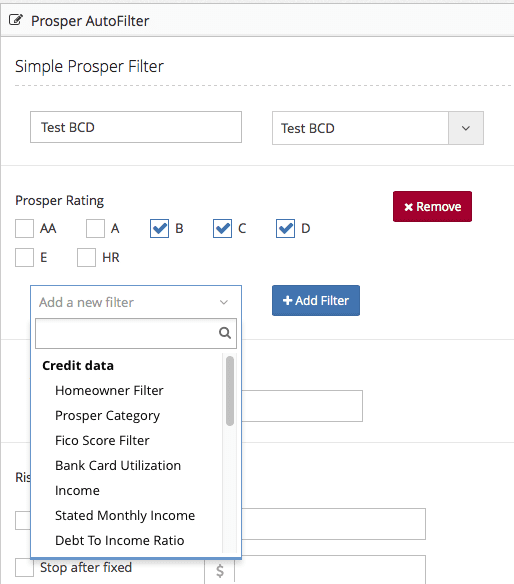
PeerToPeerQuant’s main offering is a genetic algorithm credit model for Lending Club. The model was created with the goal to determine which borrowers will pay the most to investors. A genetic algorithm is built by inputting Lending Club’s historical data. The computer program then uses this information to create investment strategies with anticipated returns. Strategies can then be combined or other criteria can be added to create better results. With an average portfolio age of 6 months, stated returns are 11.1% when calculated using XIRR. Notes using their model can be purchased by clicking “Invest” on the notes on their website. After the first free 5 investments every month, the cost is $0.15 per click.

Besides being able to purchase notes, PeerToPeer Quant offers a Percentile Calculator. This uses the data from Lending Club’s “Understanding Your Returns” page and compares your results with others. Inputting my data from my primary notes yields the below results.
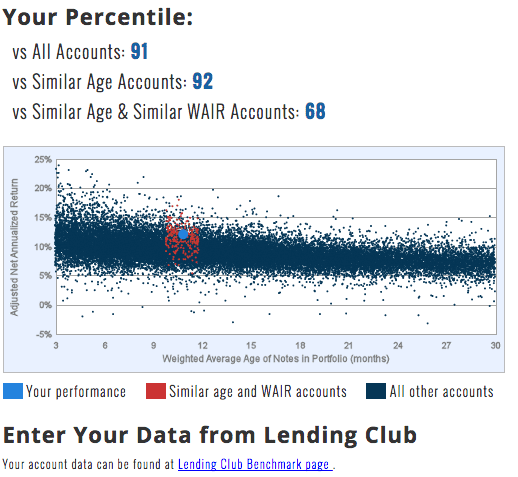
The above results show that compared to all investors, my account is performing pretty well. However, compared to those who have the same average age and weighted average interest rate, I am only in the 68th percentile. The calculator is a free tool to use and doesn’t require a PeerToPeer Quant account.
Notient is a relatively new player in the P2P lending automation and analytics space. They offer a dashboard which includes account activity, interest to date as well as portfolio breakdown. The daily charting is quite interesting, especially when gathered over a long period of time. Below you can see my account activity since I opened my Notient account.
To get started with automation, Notient offers several pre-created strategies. For instance, they have included strategies from Peter’s post on how he is investing in Lending Club and Prosper. Users are also able to create a custom strategy using fourteen attributes. From there, strategies can be assigned to auto-invest.
Notient is currently in beta and is not charging to use the platform. Looking forward, they plan to offer automated note sales and support other platforms besides Lending Club and Prosper.
InterestRadar has been around in the Lending Club automation space for quite some time and offers many different features. As a registered user, there are several public strategies that are available to choose from which aim for 10% returns. Users can edit these strategies if desired or create a completely custom filter strategy. Interest Radar has two built in scoring models to predict default which can also be used when backtesting. Both models are computed for each loan grade.
Besides investing, Interest Radar also offers an auto sell feature. This can be setup based on formulas with specific variables as well as the FICO trend. Since selling notes is a very manual process, auto sell could be useful for users who list many of their notes for sale or those who try to get rid of notes that they believe will be charged off.
By uploading your Lending Club portfolio. you have access to all sorts of insights. One of the options is “Risk”, which shows non-performing loans, loan concentration, score estimated risk and payment processing delay. You can see below that I have several notes that are deemed riskier with Interest Radar’s IR01 score (Scores range from 330 – high risk of default to 409 – lower risk of default).
A subscription to Interest Radar is $9.99/month or $59.99/year, but there is a free 30 day trial offered.
P2P Automation and Analytics Sites – Wrap Up
It is great to be a P2P investor with so many tools available. I have only scratched the surface on the functionality that these tools offer and new features will only be added as tools mature. I encourage investors to explore the wide variety of tools available and keep an eye on new ones entering this space.
What tools do you prefer and use regularly? Let us know in the comments!